Machine Learning Forecasting Development Services For Improved Accuracy By Rootfacts
Machine Learning Forecasting Applications for Precise Weather Predictions
The dynamic field of weather forecasting is an unending race for precision. However, the traditional numerical weather forecast models despite having some strengths are also faced with certain limitations. This has thus led to the introduction of machine learning into the game. RootFacts is one such company that employs it in generating sophisticated weather forecasts.
This lesson will teach you about RootFacts machine learning approach to prediction creation. Learn about machine learning (ML), how it improves performance, and how RootFacts applies it to provide users with more precise weather information.
The Importance Of Knowledge Sharing In Agriculture
Machine learning is an offshoot of artificial intelligence where algorithms learn from data without being explicitly programmed. Among these are large meteorological datasets like:
Atmospheric temperature, pressure, humidity, and wind speed measurements pictures obtained by remote sensing Radar data reanalysis (historical reconstructions of atmospheric states)
Starting at this point, those models would be able to identify minute correlations between massive amounts of input data as a result temperature measurements via-a-via barometric pressures for instance that allowed them predict future meteorological conditions.
Principal Benefits of Machine Learning in Weather Prediction
There are several major advantages that enhance forecast accuracy in relation to traditional NWP models. However:
Data-Driven Insights
Rather than relying on traditional meteorological observations only; ML utilizes other sources such as social media posts and environmental sensors.
Non-Linear Relationships
There may often be intricate interconnections rather than simple linear relations among factors related to meteorological phenomena. Consequently, conventional forecasting techniques cannot take these intricacies into account which makes them less effective at accurately predicting chaotic weather situations.
Real-Time Learning
These ML models keep adjusting themselves constantly due to receiving new data streams all time hence reacting differently towards diverse environmental situations making them become more predictive.
Feature Engineering
Traditional weather observations are not the only data inputs that we can include using machine learning. If combined, future forecasts could be more precise and comprehensive by adding environmental sensor data, crowd-sourced observations, and social media sentiment analysis.
Accelerated Processing
When producing real-time or near-real-time weather situation updates, occasionally machine learning models might be faster than traditional NWP models.
RootFacts is the top supplier of AI solutions in this area. See how they go about it:
Advanced Algorithms for Machine Learning
RootFacts uses such machine learning techniques as support vector machines, neural networks, and random forests. Each method has its own advantages based on the specific forecasting task at hand.
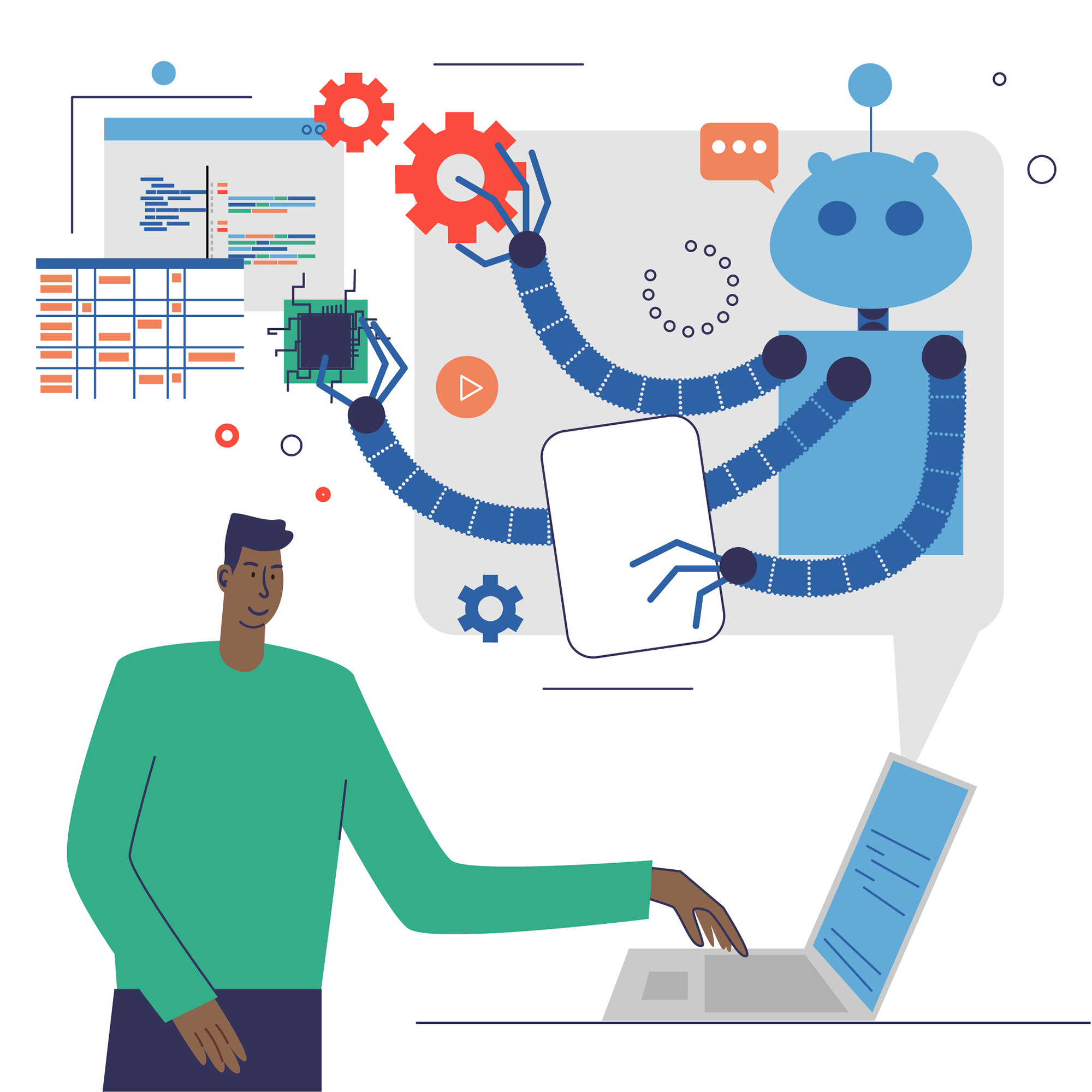
Superior Data Gathering
RootFacts considers the types and amount of data that should be used while training their models. That’s why up-to-date pre-processing and cleaning techniques along with reliable meteorological data providers are adopted for this purpose.
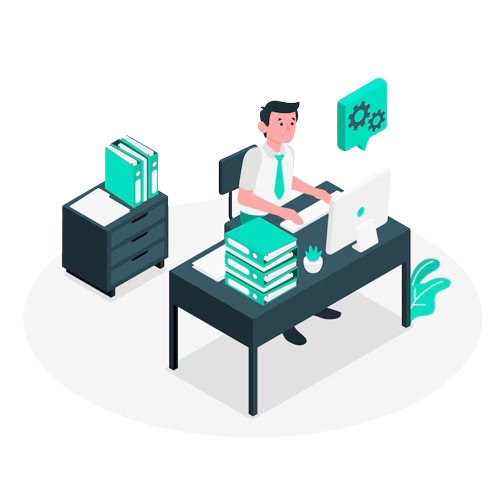
Infrastructure for Scalable Computing
ML models frequently require huge computational resources to train and apply. Therefore, RootFacts uses high-performance computing infrastructure to work with large datasets as efficiently as possible.
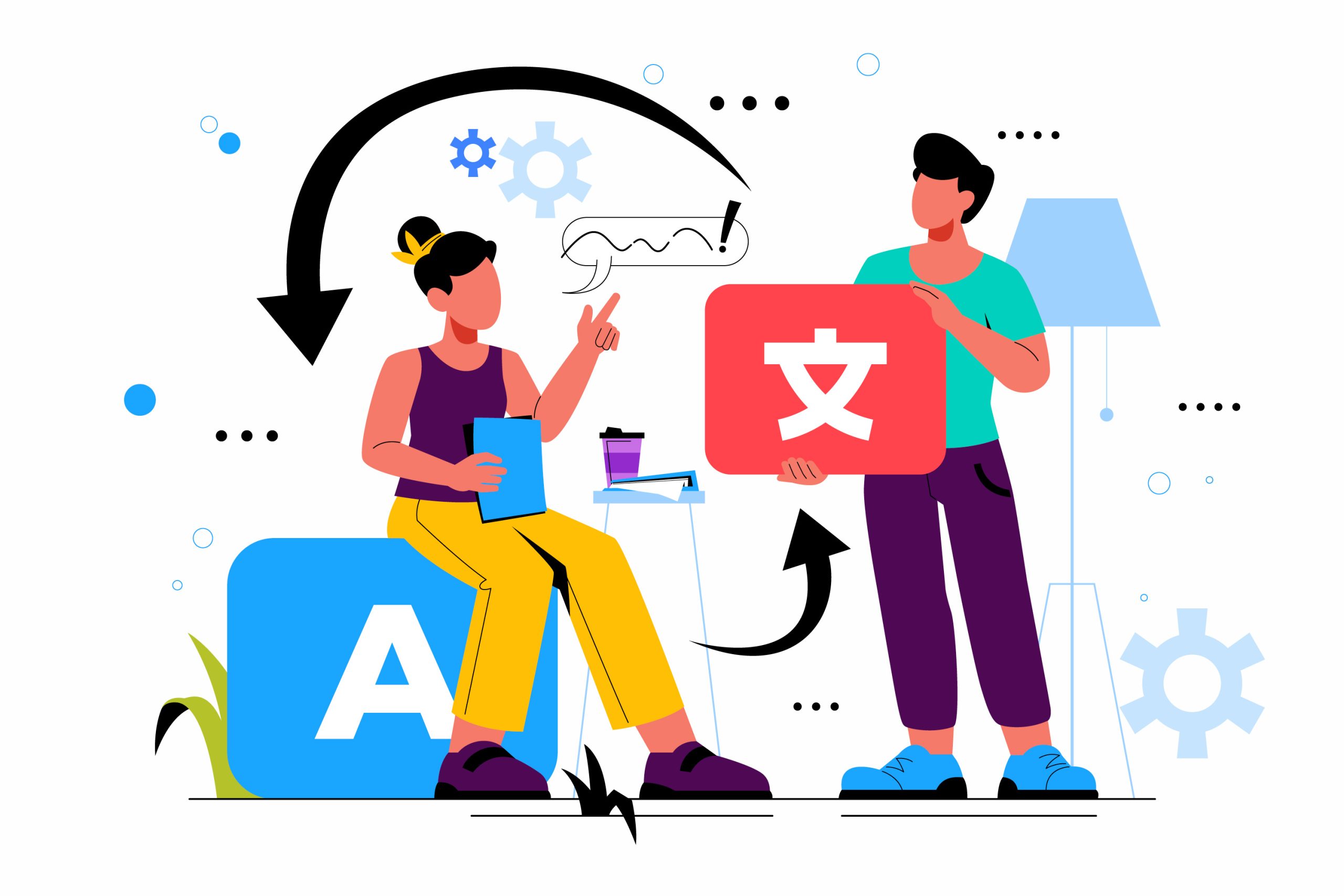
Interpretability and Explain ability
How machine learning is used by models like ROOTFACTS in producing its ML forecasts must be grasped. In addition, their concern is furthering transparency in interpretation methods used by these models.
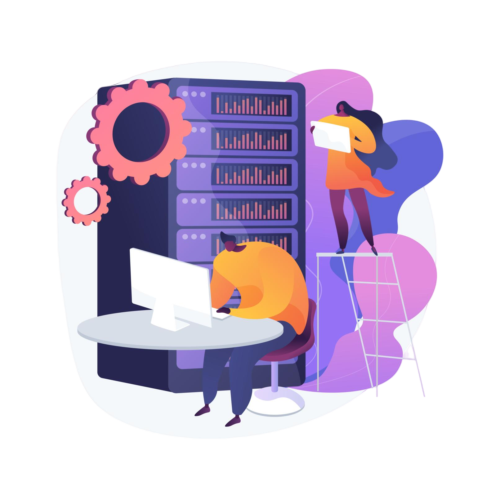
Using a human in the loop Human meteorologists are still essential for weather prediction even with the help of AI systems, as this model includes human input into the loop to improve ML systems’ recoverability. Hence, end products merge machine learning insights from artificial intelligence together with expert judgment from experienced meteorologists.
Useful Machine Learning Applications for Weather Forecasting
The following are some sectors where RootFacts employs machine learning in relation to weather:
Aviation
For poor weather conditions, airlines can forecast flights properly and thus save lives of passengers by assisting them in getting to destinations on time through a forecast created by an automated system.
Energy Sector
Energy companies can determine when best to produce or distribute electricity due to understanding climate change variables which trigger variations in demand patterns.
Agriculture
Farmers need better predictions of rainfall if they want to design planting dates well, decide when irrigation intervals will be done, come up with pest control strategies or know crop harvesting seasons accurately.
Disaster Management
Knowing what the future holds in terms of storms, floods or any other natural calamity can go a long way in helping emergency response teams to take the lead. Hence, they can allocate resources prudently and forecast threats.